Artificial intelligence | 21 Feb 2024 | 16 min
Generative Adversarial Networks (GANs) Explained
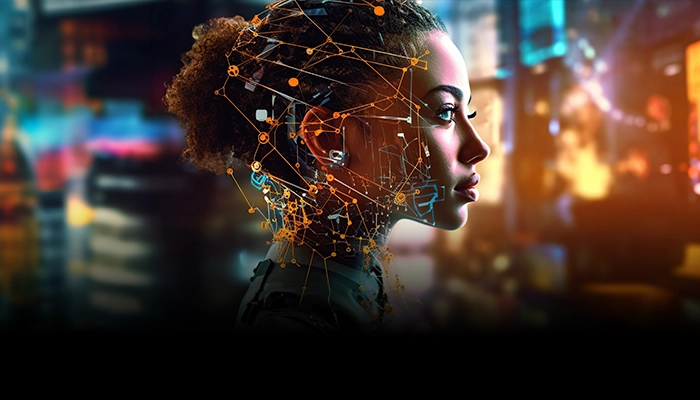
Tech-curious minds, let’s step into the fascinating world of Generative Adversarial Networks, or as they are commonly known, GANs. We’re going to break this term down into digestible pieces, see how these networks work, and explore why they are creating quite a buzz in the tech community.
Fundamentally speaking, let’s first understand what a GAN is.
Understanding the terms ‘generative’ and ‘adversarial’
Imagine you have a machine learning system that can generate images, music, or even text that look and sound like they were created by humans. That’s the “generative” part of GAN. It’s about creating something fresh, often in the form of digital content.
Time for the twist: the “adversarial” part. In a GAN, there are two neural networks at play – the generator and the discriminator. They are adversaries, always playing a game against each other.
Let’s now understand how a GAN works.
1. The Generator:
2. The Discriminator:
3. The Adversarial Game:
4. Training:
5. Convergence:
Various architectural choices influence the performance and stability of GANs. From the selection of activation functions and optimization algorithms to the design of network architectures (e.g., deep convolutional networks), each decision can greatly impact the efficacy of the model.
The architecture of a GAN looks something like this:
Fig: The GAN architecture
While GANs have showcased remarkable capabilities in generating realistic data across domains such as images, text, and even audio, they are not without challenges. Issues like mode collapse, where the Generator produces limited varieties of outputs, and training instability necessitate ongoing research efforts. Nevertheless, recent advancements such as Wasserstein GANs and Progressive GANs have addressed some of these challenges, pushing the boundaries of generative modeling.
Now that you know how a GAN works, let’s turn to the types of GANs.
Here are the types of GANs at a glance:
Fig: Types of GANs
This is the basic form of GAN, comprising a generator and a discriminator. The generator produces fake data, and the discriminator evaluates it, providing feedback to both networks to improve their performance over time.
In this variant, additional information, such as labels or attributes, is offered to both the generator and discriminator. This enables more controlled generation, allowing the GAN to produce outputs based on specific conditions.
DCGANs make use of convolutional neural networks (CNNs) in both the generator and discriminator. A convolutional neural network is particularly effective for image-related tasks, making DCGANs well-suited for generating high-quality images.
This type of GAN focuses on enhancing the resolution and quality of images. By learning from low-resolution inputs and their corresponding high-resolution counterparts, super-resolution GANs generate sharper and more detailed images.
Now you might be wondering about the places GANs find in the real world. Let’s dive into the use cases!
GANs have made immense progress in various industries. They have revolutionized processes and unlocked fresh possibilities for businesses. Take a look at these industry-specific use cases of GANs:
Fig: Industry-specific use cases of GANs
Picture the generation of synthetic medical images. This helps in data augmentation and addressing the scarcity of labelled datasets. This has improved the training of medical imaging algorithms. This, in turn, has led to much better diagnostic accuracy and treatment planning.
What’s more, GANs assist in generating molecular structures with desired properties, accelerating the drug discovery process. They synthesize novel molecules and empower pharmaceutical companies to explore a broader chemical space. This can lead to the development of more effective treatments.
In the realm of fashion, GANs have empowered designers to explore limitless possibilities. GANs train on vast datasets of clothing designs. They can generate apparel designs, textures, and patterns that will make one do a double take. This has streamlined the design process, enabling designers to quickly prototype and iterate. The result? More avant-garde collections!
GANs enable virtual try-on experiences. In these, customers can visualize themselves wearing various clothing items without physically trying them on. This enhances the online shopping experience. It also reduces return rates and boosts customer satisfaction.
That’s not all. GANs are employed to generate high-quality product images for e-commerce platforms. This allows businesses to showcase their products in diverse settings and variations. As you can imagine, this organically translates into more customers and more sales.
GANs are driving innovation in automotive design. They help out in the creation of highly realistic 3D models and prototypes. Automakers make use of GANs to generate lifelike renderings of vehicles. These allow for comprehensive virtual testing and design validation. This accelerates the product development cycle. It also reduces costs and heightens the overall design quality.
GANs have transformed marketing and advertising campaigns by enabling hyper-realistic content creation. Brands leverage GANs to generate lifelike images and videos for:
This enhances consumer engagement and drives sales. It also fosters brand loyalty in an increasingly competitive market landscape.
Yes, GANs are overhauling the gaming experience and content creation process. Game developers use GANs to generate realistic environments, characters, and special effects. These enhance immersion and visual fidelity. What’s more, GANs power procedural content generation. They dynamically come up with game levels and scenarios. All this, to provide endless gameplay possibilities!
Let’s now turn to the advantages and disadvantages of using GANs.
We can see that GANs are effective in coming up with superior data samples.
They have been found to be more stable when compared to non-relativistic counterparts.
They have also been successfully creating plausible high-resolution images from small samples.
Still, GANs have some limitations.
One real concern is the privacy implication of deep learning. Centralized training algorithms could mishandle sensitive information.
What’s more, GANs can be susceptible to attacks, like the one that uses the real-time nature of the learning process to make prototypical samples of private training sets.
All said and done, GANs do offer major advantages in coming up with high-quality data, but their privacy and security vulnerabilities are food for serious thought.
Well, it’s time for me to wrap up the ideas in this blog…
Generative Adversarial Networks represent an exciting frontier in artificial intelligence, offering endless possibilities for creativity and innovation. Whether it’s generating lifelike images, enhancing photographs, or even composing music, GANs continue to push the boundaries of what AI can attain. So, the next time you encounter astonishing artwork or seemingly realistic photos, remember, there might be a smart AI algorithm behind it, engaging in its own creative battle of wits!
As technology continues to evolve, who knows what other marvels these networks will come up with?
If you’d like to learn about the basics of generative AI, head over to this blog.
Write to us at Nitor Infotech with your views about this read. Visit us to know more about what we do as an Ascendion company.
we'll keep you in the loop with everything that's trending in the tech world.